Foundational data considerations for generative AI
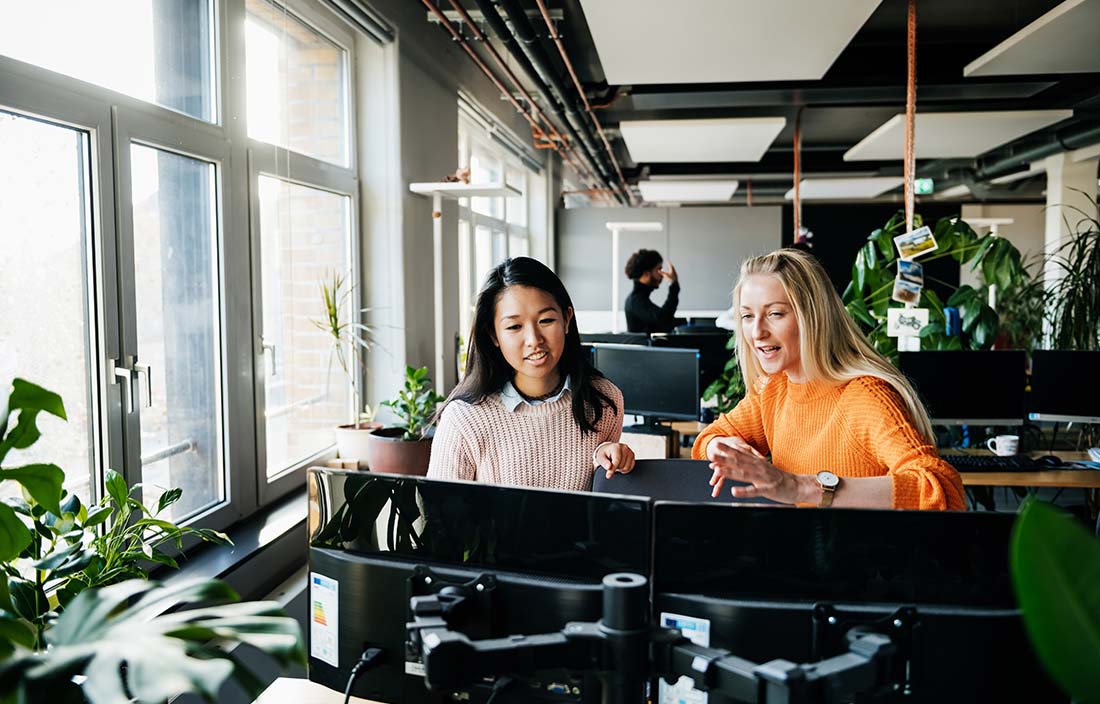
What are the key data considerations for implementing AI?
Along with the excitement at what generative AI can do, it’s important to acknowledge the hype. This isn’t unique to AI; exuberance accompanies the adoption of many new technologies. In Gartner Hype Cycle terms, this is known as the “peak of inflated expectations.” Technology A is the next cool thing, and the perception is you must jump in or risk falling (quickly) behind.
But what if you think about generative AI as you would any other tool in your IT environment and ask how you can best use it to further your mission? Integrating GenAI into your organization requires focus on core fundamentals and best practices for your data, and this focus helps you avoid getting caught in cycles of hype and fear. This will help you realize the benefits you seek from generative AI and see a return on your investment.
Data is the steppingstone to generative AI, and preparation is critical. For example, generative AI won’t fix “ROT” data (information that’s redundant, outdated, or trivial), and integrating AI into operations isn’t a shortcut to or easy button for good data practices.
Data is the steppingstone to generative AI, and preparation is critical.
Generative AI and data strategy
Does your organization have a data strategy? If not, developing one is the first step.
A comprehensive data strategy helps your organization manage, process, and leverage data as a strategic asset. In the context of generative AI, data strategy is essential. It ensures the AI applications you plan to use are fueled by well-organized and up-to-date information, allowing the system to understand, interpret, and generate high-quality content and insight that support decision-making and innovation. Put another way, it’s difficult to leverage AI if your data is siloed and you’re running shadow systems of sticky notes and spreadsheets.
If you already have a data strategy in place, you’ll want to revisit it to prioritize data quality and data readiness before considering or implementing generative AI.
Data quality and data issues with AI
Data quality is the cornerstone of a strong data strategy. For generative AI, the requirement for high-quality data is even more important. Data that’s free from errors, inconsistencies, and biases is crucial for training AI models — and for generating outputs you can rely on for decision-making. Poor data quality can lead to incorrect, biased, or unreliable outputs that can disrupt business operations or cause ethical and legal issues.
Under the hood, generative AI is a series of probabilities predicated on large amounts of data, which means the better your data quality, the more valid the outcomes. If this sounds like the old data adage, “garbage in, garbage out,” it is.
Data governance, data culture, and AI
We’re often asked about the role data governance plays with AI, including GenAI. In short, effective data governance is the linchpin to ensuring data quality. Data governance policies, procedures, standards, and clear organizational roles and responsibilities help maintain data accuracy, consistency, security, and compliance. As organizations give greater consideration to using AI, reevaluating data governance frameworks is key to ensuring data readiness for transformative GenAI applications.
Discussions about data governance must include data culture. We hear frequently from data analysts and business users who say their organizations are data-driven but data-starved, with data access controlled to the point of being nearly inaccessible. This makes it difficult for people to do their jobs day to day, and it leads to a proliferation of workaround systems, which exposes the organization to cybersecurity concerns and other risks.
We also often see enterprises with data policies developed years ago and that haven’t been documented or updated to include new tools and technologies. Equally unhelpful, we see critical institutional knowledge — e.g., organizational processes — left undocumented, doomed to be forgotten. None of these examples is a technology issue — they’re issues of data culture, and it can hold your entire organization back. Data that resides in the same place and is well-secured and accessible to an organization’s applications and people is what makes the magic start to happen.
Data governance looks different for every organization. The trick is to make it operational. Technology must be both fit for purpose and fit for use — by your staff and executives, your customers, your community. And this requires asking: What are we trying to solve, and how can AI — or any new technology, for that matter — help?
Data readiness for generative AI
Data readiness for AI will be the differentiator between stagnation and competitiveness. Generative AI is early in its journey and it’s evolving rapidly, which underscores the need for a solid foundation — data strategy, data quality, data culture, and governance. The right data environment will be paramount to harnessing the transformative power of GenAI as it continues to evolve and to positioning your organization to thrive.
Data readiness for AI will be the differentiator between stagnation and competitiveness.